
Steven Lee
Where there's a will, there might need to be a new scientific way
For every tidbit of information that textbooks are able to state as a matter of fact, researchers have spent months, or even years, uncovering them using intricate and often clunky scientific tools. A whole day of running electrophoresis gels, for example, can separate out different-sized pieces of DNA as they snake through a web of agarose; and particular staining and imaging techniques can reveal structures and processes invisible to the naked eye. These techniques become the natural filter through which many biomedical researchers approach a problem -- how can this question be broken down, they ask, so that we can solve each part of it with the tools we have available?
But there are times when the techniques scientists have at their disposal can’t quite get to the heart of the question. When this happens, researchers face a choice: they can either regroup and come at the problem from a different direction, or they can develop an entirely new tool. Recently, researchers at Whitehead Institute have been busy developing, testing, and writing about new methods they have developed to tease out answers to their questions about what is going on in tumors, viruses, and human gene expression. From mice with human nervous systems to an algorithm that can determine the shape of RNA, we’ve collected a few of our favorite new ways to study some of the most intractable and perplexing questions in biology.
A nervy mouse model
Neuroblastoma is a rare cancer that affects around 800 children in the United States each year. The disease is notoriously hard to study for a number of reasons. For one, it often develops in utero, making it nearly impossible to understand how it starts. For another, the cancer has very disparate outcomes -- in some cases it resolves on its own, while in others it is much more aggressive and often fatal.
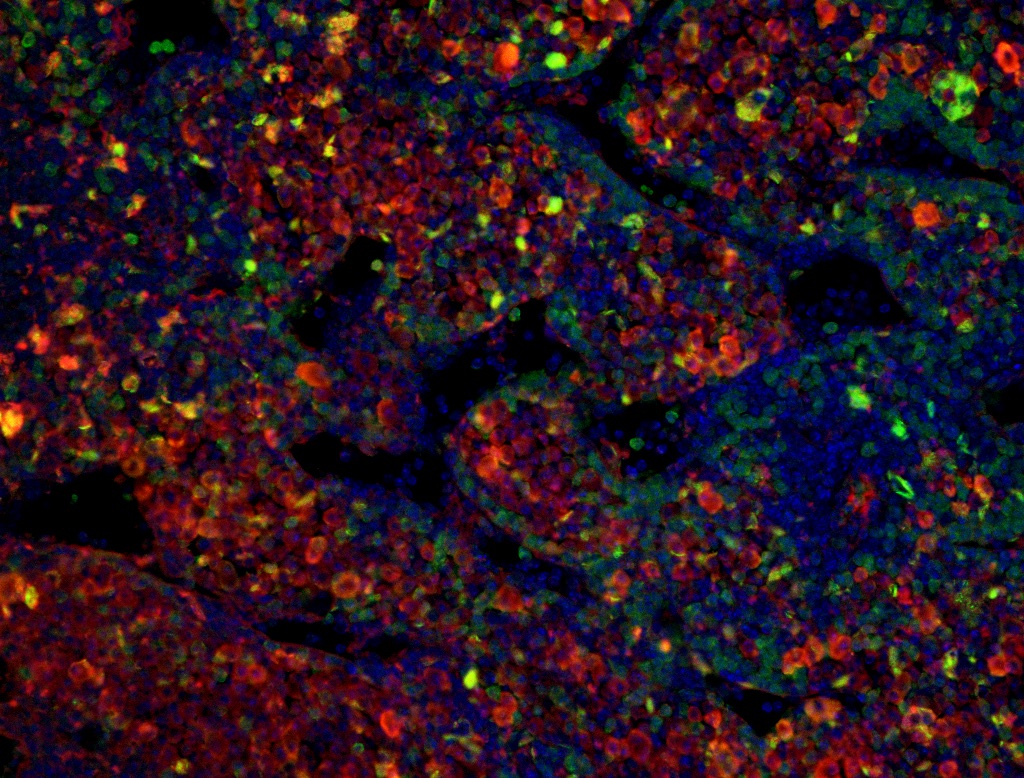
In the past, researchers studied the cancer by inducing tumors in mice. However, these mouse cancers could only go so far in explaining the behavior of human ones. That’s why Malkiel Cohen, a former postdoc in the lab of Whitehead Institute Founding Member Rudolf Jaenisch, wanted to find a new way of modeling this disease. Jaenisch and his lab had been working for years to create chimeras, or organisms that contain cells from another of a different species, to use as models in such situations; if this model could be tweaked a bit, Cohen thought, it could be an exciting new way to study neuroblastoma.
To begin, Cohen obtained human stem cells and modified them to express two genes known to be associated with the cancer. Cohen then coaxed the stem cells to differentiate into neural crest cells, the cells from which human neuroblastomas are derived, by injecting them into the neural crest tissue of mouse embryos. He then watched over the following months as the cells proliferated and crept into the nervous systems of the growing mice: they were now ready to model human cancers. To test whether the model would work for neuroblastoma tumors, Cohen treated the mice with a chemical that would induce the cancer, and studied the ensuing tumors.
The tumors ended up being quite similar to human tumors, both in their structure (some were rosette-shaped, just like in many human cases) and in their genetic makeup. One key similarity was in the way the neuroblastomas affected the mice’s T cells -- a type of immune cell that recognizes cancer cells and attempts to destroy them. Neuroblastoma tricks the T cells by activating a chemical signal that exhausts them and weakens their attack.
“The obvious next step is to study how treatment of these tumors will allow these chimeric mice to be cured,” Cohen says. “This is a model that will allow us to approach not only how to get rid of the tumor, but also to fix the immune system and recover those exhausted T cells, allowing them to fight back and deplete the tumor.”
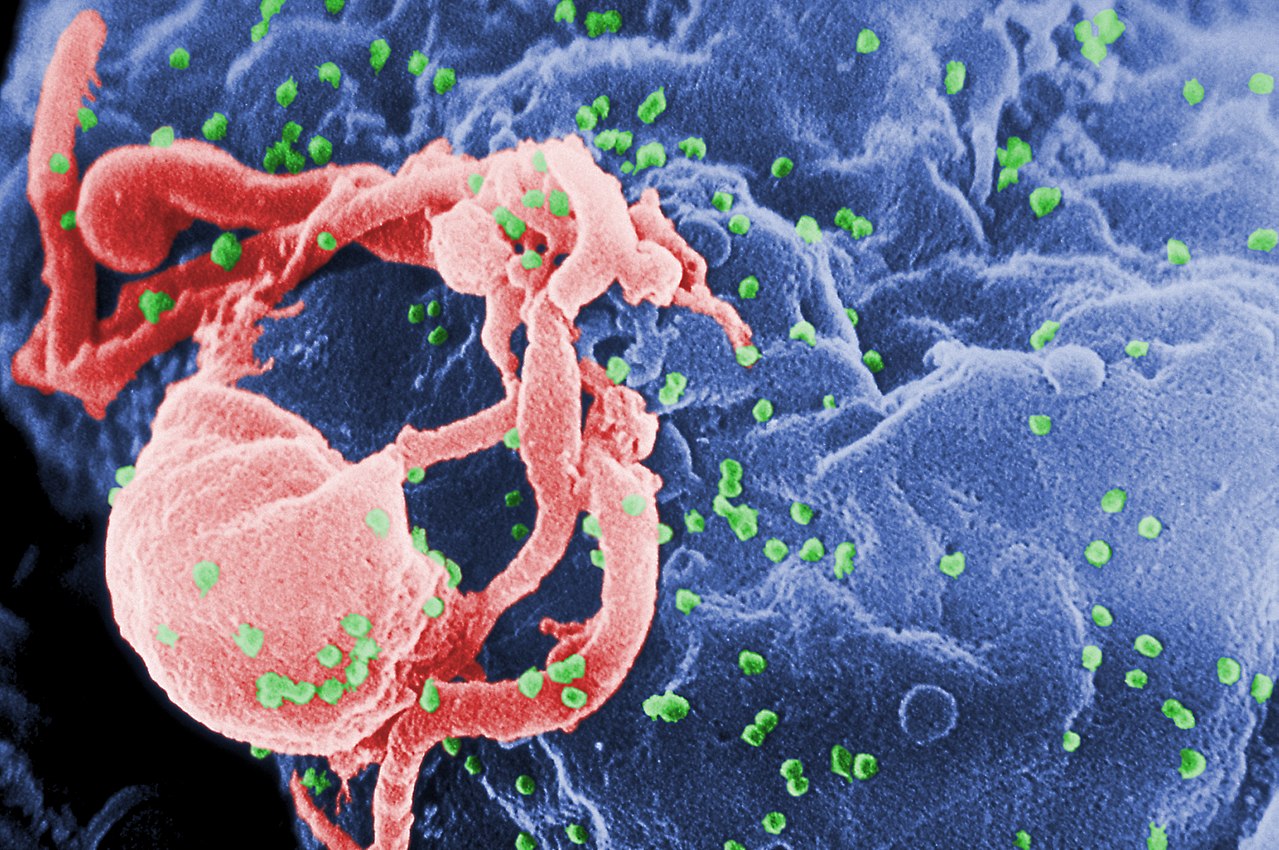
Big DREEMS for understanding RNA shapes
For all the devastation it can wreak on the human immune system, human immunodeficiency virus (HIV) sure is tiny: the virus, which causes AIDS, only has nine genes from which it creates every protein it needs to take over an entire cell. To make these proteins, it hijacks its host’s molecular machinery to snip its RNA transcripts into protein-coding pieces. Whitehead Institute Fellow Silvi Rouskin wanted to know how the tiny virus was able to direct how it was cut up. She had a hunch that the shape of the RNA molecules had something to do with it. Maybe, she hypothesized, the RNA molecules controlled where they were snipped by crumpling into different conformations.
But the existing methods for measuring the shape of RNA were not precise enough to draw any conclusions, and came with a couple of caveats. For one thing, they relied heavily on theoretical methods -- for example, calculating the likely shape of an RNA sequence based on thermodynamic laws -- and they usually operated on the underlying assumption that pretty much every RNA molecule with the same sequence was shaped the same. In order to test her hypothesis, Rouskin needed a method that didn’t make any such assumption.
Enter a new method: the DREEM algorithm. Rouskin tagged the open bases (those not bound to other bases on the molecule) on RNA molecules with chemical markers, which led to mutations when the RNA was converted to DNA.
Rouskin created an algorithm that could determine multiple shapes the RNA can make in a cell by analyzing which bases were “open” -- that is, not bound to another part of the RNA molecule. The algorithm could also estimate the relative frequency of those shapes. Rouskin and her colleagues tested the algorithm on a piece of human RNA that was known to have two different conformations, and found they returned accurate results. When they put it to work in HIV genomes, they found that the shapes of HIV RNA are much more variable than previously thought.
Rouskin’s new method has the potential to help scientists learn more about human RNA as well. “People have learned a lot of things from viruses and then realized that the human cell is doing the same thing,” she said. “We're very excited.”
Genetic variants on the brain
In multiple sclerosis (MS), the body’s immune system attacks its own nervous system, destroying the protective sheaths surrounding the nerve fibers in the brain. This results in symptoms such as loss of vision, pain and fatigue, trouble walking, and sometimes the loss of basic functions.
Environmental factors like viral infections and tobacco smoking, as well as several genetic variants -- regions of DNA that are different from person to person -- have been shown to be associated with the disease. Still, scientists have not yet pinned down exactly how they all work together to create the devastating condition.
Olivia Corradin, a Whitehead Institute Fellow, was interested in how the variants were related to the disease: what effects were specific variants having on cells that increased patients’ likelihood of developing MS? Previous methods analyzed the variants as a group -- kind of like a big pot of soup -- but Corradin knew this wouldn’t give her the answers she sought. So she created her own tool to examine particular ingredients of that soup, and find out which cell types were being affected by individual variants.
Her technique, called the outside variant method, considers not just a single varying sequence, but also how these MS-associated variants interact with other DNA regions that fold together inside the cell. She identified DNA variants that are close in 3D space even though they may be far apart on the chromosome, which she called “outside variants.” She then looked at whether people that had these groups of variants were more at risk for MS than others. When they were, she looked for cell types that needed to use these DNA regions for important cell type functions.
As expected, these variants played a key role on the functions of immune cells such as T and B lymphocytes. But the approach also turned up something new: Corradin found evidence that MS-associated genetic variants can affect the brain cells responsible for producing myelin, called oligodendrocytes. This is the first time researchers have found evidence for MS variants affecting brain cells. The knowledge of how MS works in these cells will help doctors and researchers better understand the progression of the disease, and will inform efforts to develop better treatments.
And Corradin’s outside variant method will likely help more people than just MS patients; in the future, she plans to use the outside variant approach to analyze other diseases that result from combinations of genetic and environmental factors, such as cancer, heart disease or psychiatric disorders.
Predicting the behavior of some of the cell's smallest RNAs
Cells don't make proteins out of all their genetic material all the time. In fact, the differences between the various cells throughout the body (e.g., the cells of the skin versus those of the brain) are largely due to the different amounts of each protein being produced within the cells of each tissue. Cells have numerous mechanisms to control how much protein is made from each gene. One such strategy involves short RNA molecules called microRNAs.
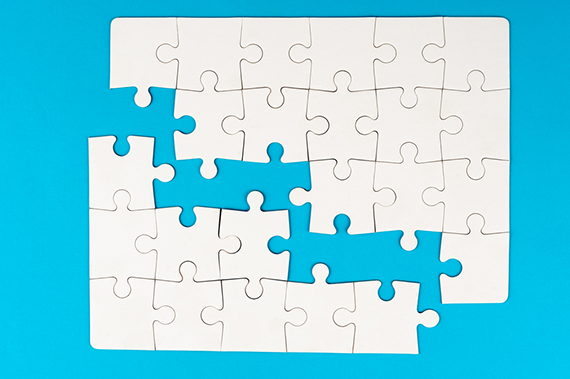
These tiny molecules—only about 22 nucleotide building blocks long—bind to specific sequences within the cell’s messenger RNAs (the RNA molecules that are translated to make proteins), or mRNAs, and cause the cell to degrade these mRNAs more rapidly than it does ordinarily. This lowers the total amount of the corresponding protein made by the cell.
Each of the hundreds of known animal microRNAs are better at repressing some mRNAs over others, and knowing what causes this variation would help researchers better understand a cell’s regulatory network as a whole. However, predicting the targeting preferences of a microRNA is challenging: many mRNAs are repressed significantly more or less than predicted by researchers’ calculations. In the past, models used to predict microRNA behavior were based on the average behavior of many different microRNAs, because there simply wasn’t a good way to look at individual molecules one by one.
That’s where Whitehead Institute Member David Bartel and his colleagues came in: Bartel, graduate student Sean McGeary, and former graduate student Kathy Lin developed an experimental and computational approach to test how well an individual microRNA binds to a vast number of different RNA sequences. Bartel is also a professor of biology at the Massachusetts Institute of Technology and an investigator at the Howard Hughes Medical Institute.
By applying their new technique to six specific microRNAs, the researchers uncovered unexpected differences in the behavior of these tiny molecules. In particular, they found that how strongly a microRNA binds another RNA sequence is not well explained by prior models of how nucleic acids interact—for example, an exact match-up of base pairs was not as important as previously thought. In addition, the interaction between surrounding bases could lead otherwise preferential binding sites to be less accessible to the microRNA. Faced with this unexpected complexity, the team used machine learning to build a model that captured these features as well as any hidden patterns within the data.
The researchers will use their findings to make major updates to an online tool called TargetScan, which Bartel and the Whitehead Institute Bioinformatics and Research Computing group developed and currently maintain. TargetScan enables researchers to assess whether a gene of interest is likely regulated by any microRNAs. For example, a neuroscience researcher studying the neurotransmitter glutamate would learn that the mRNA corresponding to the glutamate receptor (GRIA1) is likely targeted by microRNA 183 and microRNA 96, among others. This will enable the results of their study to provide further, continued benefit to the biomedical research community.
A little love for new scientific techniques
With a few exceptions, the papers that these novel tools appear in aren’t about the tools themselves. The findings of the study often stand on their own -- and if you’re not paying attention, it can be easy to take the results of the study and forget the other important finding: a brand new method that can help future scientists in their searches to comprehend the complexities of biology.
***
Written by Eva Frederick
***
Sources:
Cohen, M., et al. Formation of Human Neuroblastoma in Mouse-Human Neural Crest Chimeras. Cell Stem Cell. March 5, 2020. DOI: https://doi.org/10.1016/j.stem.2020.02.001
Factor, Daniel C. et al. “Cell type specificity of intralocus interactions reveals oligodendrocyte intrinsic mechanisms for multiple sclerosis.” Cell, April 3, 2020. doi: 10.1016/j.cell.2020.03.002.
Tomezsko, P. et al. Determination of RNA structural diversity and its role in HIV-1 RNA splicing regulation. Nature, May 6 2020. doi:10.1038/s41586-020-2253-5
McGeary, Sean E. and Lin, Kathy et al. The biochemical basis of microRNA targeting efficacy, Science (2019). DOI: 10.1126/science.aav1741
Topics
Contact
Communications and Public Affairs
Phone: 617-452-4630
Email: newsroom@wi.mit.edu